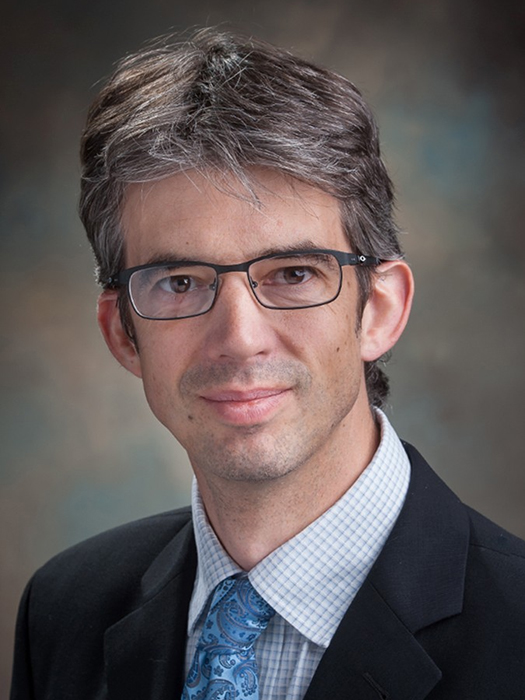
This episode features our conversation with Roger Linington, from Simon Fraser University. Roger is a natural products chemist, and his research group works in metabolomics, drug discovery and screening, structure elucidation, and chemical biology. In recent years, his group has turned to some software development in order to build the tools that they need, and The Natural Product Atlas, a high-quality freely-available natural products structure database is one great result. In our conversation, we talk about NPAtlas, and the large international collaboration that produced it, what one can do with all that information, and we muse on how to start connecting the large and ever-growing set of omics data that natural products research continues to produce.
DAN: Welcome back for episode 9 of Natural Prodcast. This week, Alison and I talk to Roger Linington, from Simon Fraser University in British Columbia, Canada. This is the last of the podcasts we recorded in my hotel room at SIMB, or the Society for Industrial Microbiology conference that took place in January this year in San Diego, and that city is where I first met Roger, when we were both postdocs at Scripps Institution of Oceanography. Roger was splitting his time between Bill Gerwick’s lab and doing research in Panama through support from the ICBG training program, which stands for International Cooperative Biodiversity Groups, and it’s this great program funded by the National Institutes of Health and the National Science Foundation to create international partnerships in developing countries for research into various aspects of biodiversity, of which natural products chemistry is one.
DAN: So, I’ve always known Roger as an expert in natural product structure elucidation, but more recently, he’s been leading an effort to build The Natural Product Atlas, or NPAtlas, a freely accessible natural products structure database, which is going to be just invaluable to the natural products community. It’s a big international community effort, and I want to say thank you, personally, to everyone involved in making this happen. We had a really fun conversation with Roger about all this, in terms of how to build an international community collaboration, and in thinking about the future of the natural products field as it relates to structure elucidation and genome mining, and how we might start to better connect those two things, because I think that’s ultimately what needs to happen if we’re really going to understand the chemical diversity and chemical utility that nature has to offer us. I found this conversation really inspiring, and I’m super happy to be able bring it to you. You can access NPAtlas right now at npatlas.org, and we’ll have links to it in the show notes at naturalprodcast.com.
DAN: I also just want to say thanks to everyone listening. Our download numbers keep going up, which continues to surprise and amuse me. I can’t tell, of course, whether this is just the natural products community listening to itself talk, or if we’re finding an audience outside of just us. If you’re new to natural products science, please let me know! I’m dying to hear from you. Toss an email to [email protected], or leave us a review on Apple Podcasts or wherever you’re getting this and tell me why you’re listening.
DAN: But, now, here’s Natural Prodcast Episode 9 – our conversation with Roger Linington.
DAN: Hey, Alison. We’re still at SIMB.
ALISON: Yay! SIMB!
DAN: We have one more interview to do at SIMB. And sitting in the crazy hotel chair now is Roger Linington. Roger is a professor at Simon Fraser University. And I have known Roger for quite a while. We met right here in San Diego, at Scripps [Institution of Oceanography].
ROGER: Yeah, that’s right. We were postdocs together in the mid 2000s, I guess. You were working for Brad Moore. I was working for Bill Gerwick as part of the International Biodiversity Drug Discovery consortium doing natural products isolation/structure elucidation type work.
DAN: Yeah, that’s right. I want to talk about that – what were the letters? IBDD?
ROGER: ICBG. It’s the International Cooperative for Biodiversity Groups program, a really amazing program, funded by the National Institutes of Health.
DAN: All right, why don’t you tell us a little bit about that.
ROGER: Yeah, this was an amazing program. So the program is still running. And its premise was to partner US institutions interested in natural products, with institutions in developing nations to do natural products based drug discovery, predominantly in diseases of importance to each host country. It was built very much on the foundations of sort of cooperative and collaborative science. It was designed to ensure equitable benefit sharing if any discoveries were made that were of value to the host country. And it had a huge mission to perform technology transfer and infrastructure development in these host countries. So, yeah, it was an amazing program.
DAN: Yeah, a lot of really great academics came out of that too.
ROGER: Many academics came out of it. Many scientists were trained on both sides – on the US side and on the host nation side. So there were many of these, with different groups. Our one was partnered with institutions in Panama. And so I had this very unusual postdoc where I went to Panama, straight out of my graduate school, and was given a small lab in the National Science Center there and ran this sort of semi independent research program, as part of my postdoc. It was great fun and did lots of fun science.
DAN: Yeah, so let’s let’s rewind in time a little bit and, before that, what got you into natural products in the first place?
ROGER: So I was originally trained in the UK, I did my undergraduate degree at University of Leeds. And there the focus was very much on sort of synthetic organic chemistry and sort of classical divisions in the chemistry landscape. So I was very much an organic chemist and I thought that I would become a synthetic medicinal chemist.
DAN: Yeah, a lot of us start that way.
ROGER: Yeah, it’s a common track.
DAN: At least, the chemistry oriented people, yeah.
ROGER: At that time, the UK didn’t have a particularly strong emphasis in natural products, certainly not in the school that I did my undergrad degree in. But I worked – as part of the undergraduate degree, there was a chance to do a year in industry. And in many ways, that was the best part of my undergraduate training.
I got to work for Pfizer, who at that time, had a big facility in the south of England. And that facility included both European scientists and North American scientists. And I saw a very different viewpoint between those two groups and the ways in which they approached scientific problems. And so that sort of opened my eyes to the idea that you could go internationally to do the next stage of your training and that there might be more to be gained than just subject matter, by making that change. So I went to the University of British Columbia, in Canada, to do my PhD. And there I was exposed to many more themes surrounding natural products. And so that sort of really captured my attention. And so I worked for Ray Andersen there, and had a very enjoyable time learning about natural products science.
DAN: Yeah, great. So Pfizer was doing natural product based drug discovery, then?
ROGER: They did still have a natural products program. But again, in the European side, we didn’t hear much about that. That was very much medicinal chemistry. My job was to make a molecule a day. And one of the things I wanted from that experience was the chance to see what the industrial life was like. And I think I learned that I’m more of an academic than an industrialist…. But that’s good! I mean, I think that one of the many good things that comes out of those kinds of training experiences is the chance for you to test the waters and see what it’s really what it’s really like behind the scenes. So yeah, I’ve benefited enormously. I’m not sure how much Pfizer benefited from my employment but I got a great deal out of it.
ALISON: I am curious to hear a little bit about what you … What you saw in the different perspectives that people trained in North America brought to the UK?
ROGER: Yeah, it’s difficult to generalize, of course, but I felt overall that the British approach at that time was quite linear and that people would take problems. And they would take a sort of foundational starting point. And they would work linearly through all of the possibilities until they reached some conclusion about that problem, whereas the North Americans tended to be slightly more out of the box. So they would approach the problem sometimes from very unexpected orthogonal perspectives. And sometimes one of those approaches would work more, would be more successful, and then other times the other one would. But I had come from a very linear background, like, the training I had received had been quite linear. And so I liked the idea that it was valuable to read broadly and think broadly about problems and to be open minded about other ways in which you might address or solve those problems. And so I hope that I might get more exposure to that here in North America.
ALISON: Yeah, and it does sound like your career has incorporated a lot of diversity. I mean, going to Panama, as well. And then also Canada.
ROGER: Yeah, there’s been geographic diversity, I’d say perhaps more interestingly, though, there’s been quite a lot of thematic diversity. So when we first started out as a research lab, we were also quite focused on finding bioactive molecules and doing medicinal chemistry, doing development of projects on a project-by-project basis. And the more time has gone on, the more we’ve become interested in sort of systems level approaches. So rather than asking one question about one class of molecules or one particular target, we’re now very much – we’re interested in how we can build tools which will let us see the whole landscape of natural products. So you know, for example, what are all the compounds in a sample set, and how are they distributed? And that’s been a sort of evolving trajectory. I think, you know, most scientific careers are like that, in that they they follow an arc. And so, you know, definitely there has been a strong evolution in terms of the way the group thinks and that the kinds of problems we’re interested in tackling.
DAN: Yeah, that that is the main reason I wanted to get you in the gaudy hotel chair today is to sort of talk about that direction of things. Because I think there’s a lot of this going around now, which I haven’t seen in natural products a whole lot in the past. This sort of idea of, “there’s a lot of data out there: let’s put it together”. So you have – at least you’re the corresponding author on the NPAtlas effort.
ROGER: That’s right.
DAN: So I know there’s a lot of people involved in this. It’s a big community and you should name them all right now off the top of your head.
ROGER: I don’t know that I can list them all! So there are … You’re right. So this is a big, community driven collaborative effort that we’ve put together to try and create an open source database of all known microbial natural products. So if you think about natural products science as a field, we have arguably been studying microbial natural products in an ordered way for perhaps 80 years. And in that time, we have discovered many thousands of different natural product classes and tens of thousands of members of these classes. And yet, there is no central open repository, which contains all of that information.
In other words, we have invested an enormous amount of time and resources and money in studying this field. Yet we don’t really know what we know. The information is scattered throughout literature. It’s across a huge array of different dates, journal titles, languages, fields – it’s really a bit of a mess. And many of the system wide tools that people would like to build would greatly benefit by knowing what’s already known. So you can imagine, if you have a particular sample, and you don’t have such a database, then if you want to know its identity, you really have no idea. You have to start from ground zero again in order to solve what might be an already known compound.
ROGER: However, if you had a comprehensive list of all the things that have previously been found, the first question you should ask is, is it one of these? And there you’re going from a question which is essentially boundless to a question which is very well bounded. And so there are plenty of strategies one can use to compare existing data to a new data set. But you have to have those data in a reasonable format. So that was our main motivation. So it is an altruistic effort in that it is a database which is now shared with the whole community in a completely free and open manner. But it is also self-centered in that we want that data set in order to do some of these projects on our own. And our motivation for starting the project was that we felt hamstrung by not having that information available. And so that’s sort of what, what motivated us to do it. Of course, it’s pretty complicated because what you’re effectively aiming to do is to graze across all of the published literature for the past hundred years and identify every article which describes the discovery of a microbial natural product –
DAN: Right.
ROGER: And that’s a pretty tall ask. So this has been a big community effort. We’ve had tremendous support from lots of researchers around the world. Europeans and North Americans, folks from South America – all over the place. And we would never have been able to do this without that sort of consulting effort. So, you know, it’s maintained by us, but it’s built by the community. And I think that’s something we all should be quite proud of, actually.
DAN: Absolutely. How do you get started on building a collaborative community like that to do such a big job?
ROGER: Yeah. So we were a little overwhelmed by the project at the beginning. It wasn’t really clear how best to begin. And so in the end, we did it in several stages. So we started out internally by making a list on the whiteboard of the top 50 journals that we thought were most likely to contain natural products articles. And then we acquired the titles and abstracts for all of the articles from all of the issues for those 50 journals. For – [it] varied -something like 20 years, and then we – I – forced the lab group to take a week off from lab chemistry research, and to go through all of these abstracts and titles and to fish out data about new molecules that have been found. And that initial work was very labor intensive. But it gave us a basal training set we could use to build more sophisticated tools, because now we had a very reasonably large set of articles which had been manually curated for whether they were or weren’t about the topic we cared about. And that gave us about 12,000 molecules. And that was enough for us to build a basic database structure and a basic web interface. And we could then show that to collaborators and say, “This is a prototype. If you will help, we could do this in a more sophisticated way.” And that was enough to capture people’s attention and convince them it was worth putting that time into.
ALISON: What was the role of potential collaborators? Like, what did you pitch to them that they could do?
ROGER: So we made a fairly mercenary trade with the collaborators. So, we used those initial analyses we’d done by hand, in order to build a machine learning model that would look at all of the data that’s in PubMed, and pick out articles which the system considered to be likely to be about microbial natural products. And then we used a bunch of text mining tricks to try and extract all the relevant data from each title and abstract and find structures that went with those. But we needed somebody to look at each one of those and curate the information and make sure that what the algorithm had done was actually accurate. And so we told people that if they would curate 1000 entries, that that would earn them authorship of the study when it came out. And that gave us enough coverage that we could ensure that most entries were looked at more at once, and so the quality – the data quality – was reasonably high. And yeah, it gave it gave us enough enough pairs of hands to be able to get through all the all the entries
ALISON: And how many entries are there now?
ROGER: There are currently just over 25,000 compounds in the database. We don’t know exactly how many there are to find. Conservatively, we estimate perhaps 40,000. The best we can do is take sections of the literature we’ve already curated, and then go through them manually to see what we missed. And from that you can get some kind of projection for what’s yet to come. So there are lots of tricks we can do to try and backfill the legacy data. Some of that’s quite difficult because styles have changed a lot over the years. So the ways in which people write have changed. And so that means that your text matching machine learning model does better with more modern articles than it does with older articles. Some of the older articles aren’t digitized. And so that makes them hard to access. The articles – the structures in older articles – are presented in quite unusual ways sometimes. And some of the really early work – it took a long time for .. I mean, structural elucidation was extremely difficult before the advent of two dimensional NMR and high resolution mass spectrometry. And so some of those stories percolate through the literature for a long time before researchers reached consensus on structural identity. And so those stories can be very laborious to curate, because you end up having to sort of walk through a lot of historical literature until we find the place where the structure was first proven.
DAN: Yeah. You wonder how many of those old structures are just flat out wrong, too, right?
ROGER: Yeah, so we do have an ongoing effort to try and capture reassignments, and many of those come from efforts with total synthesis or re-isolation. So that is something that we are trying to put more energy into now. Ultimately, we would like to capture all the instances of total synthesis because I think not only will that draw in the synthetic organic community, but also it means that if you have a compound or a class that you’re really interested in, you have immediate information about whether or not any of the members of that class have been subject to synthesis and whether there are routes which can be used to produce analogues or to do development. And I think those kinds of things can really help people to prioritize projects and do project selection. So, yes, there’s lots and lots we can add.
DAN: So in natural products, having a large data set of structures is obviously very valuable. From my perspective, JGI has a large database of biosynthetic gene clusters and biosynthetic gene cluster fragments, and all kinds of other crazy things. What do you think the community needs to do to start connecting that data?
ROGER: I think this is a really fascinating question. I think it’s one of the unsolved questions in natural products.
DAN: Absolutely.
ROGER: If I want to be provocative, I have noticed that many talks about biosynthesis start by saying “Organisms contain many gene clusters, but very few of these have known molecules. Therefore biosynthetic gene clusters represent this untapped and cryptic world of new chemistry, which we can discover.” But as devil’s advocate, I could start most natural products discovery talks by saying, “We have found all these compounds and there are no gene clusters.”
DAN: That’s right.
ROGER: So, either biosynthesis researchers are not very good at finding compounds and chemists are not very good at finding gene clusters. Or, there is a high degree of overlap between those two data sets, which we have not yet recognized. So.
DAN: It’s one or the other, right?
ROGER: It’s one or the other, or possibly some hybrid of the two. Likely somewhere in between.
DAN: Halfway in between. Yeah.
ROGER: But at the moment, you know, most gene clusters have no product and most products have no gene cluster. So either both of those pools have to increase in size dramatically, or there has to be a significant collapsing of that overlap. I suspect the latter is more likely to be true. People have studied chemistry-first projects for 80 years, from a huge array of different perspectives. They’ve studied it from the perspective of biofouling, from chemical ecology, from drug discovery in every possible disease target area, using an enormous array of different isolation strategies and different source organisms and so on and so on. So the idea that, you know, greater than 95% of the chemistry remains unfound seems unlikely to me. That’s I mean, that’s my controversial personal perspective.
DAN: Okay. Yeah.
ROGER: So I think what’s probably more likely to happen is that we will improve our ability to make relationships between structure and gene cluster and that we will see now, fairly soon, a fairly rapid collapse in the unknown fraction on either side there between both gene clusters and products. How we do that is still quite tricky. You can’t easily do it by forward prediction from the gene cluster. Not to the discrete compound, in many cases.
DAN: In many cases. Sometimes you can.
ROGER: Sometimes you can.
DAN: But also it’s a question of labor too, because you know, I spend a good chunk of – have spent a good chunk of my life staring at gene clusters and trying to understand what the chemical products are, and sometimes you get pretty close. But there isn’t enough time, probably in the rest of my lifetime anyway, to anywhere tackle that. So you’ve got to do it computationally. Right? So where do we start with that?
ROGER: Well, I think one of the areas where we need to continue to invest effort is this question of chemical constitution. So that question would be much easier to answer if we had a ready mechanism for describing the chemical landscape of any sample set. So if you have 100 organisms, and you ferment them under one set of conditions each and you extract them all, you end up with some pool of natural products. And if we could describe even the number of unique molecules that are in that set, and how those unique molecules are distributed within the set, then it would be a lot easier to start to make connections, or at least hypotheses, about the relationship between a compound or family of compounds and corresponding gene cluster or family of gene clusters, which have sort of co-occurrence.
DAN: Right, right, right.
ROGER: That are found that way. And so relatively simple changes like that, I think, will make a really big difference in the way that we’re able to approach these kinds of system-wide problems. And it’s not just constitutional analysis as a problem, we need corresponding improvements in our ability to recognize whether or not gene clusters which don’t look that similar actually produce similar products. So I think we need advancement in sort of both areas. And I suspect that no single change will resolve the problem. But that stepwise changes on both sides of the fence will mean that at some point, the whole thing suddenly collapses and becomes very much more straightforward.
Obviously, I’m much more familiar with the, sort of, chemical analytics and identification side of that problem. And I see all sorts of exciting areas and new developments and new developments in mass spectrometry hardware, software, new developments in NMR methods, ultra fast methods for acquisition. Things like this new SMART technology from UCSD, which is doing pattern recognition in comparing spectras to one another. And community-based resources which provide large, high quality, well curated datasets upon which to base those sorts of tools. So I think you need all three of those really. And from there, yeah, eventually we’ll know the answer.
DAN: How long will that take? Obviously, it depends on how many people are working on it and the right people in the right situations. But what do you think?
ROGER: I’d be surprised if it was still a problem in 10 years. I think that the field – I would say that the field of natural products is undergoing a really exciting Renaissance, as tools and opportunities in other research areas come to bear on the field of natural products. I feel that natural products science, perhaps, lagged in innovation during the tail end of the, sort of, structure-first individual compound discovery phase. And that there was a bit of a plateau there where many groups knew how to do “isolate and elucidate”-type projects. And so, now, we’re seeing this sort of new generation of tools come along, which provide a much broader view on natural product science. And I think that that’s opening up opportunities across the board. And because of that, and because of the rate at which that’s changing, I think that it’s a really exciting time to be a natural product scientist. It’s, it’s like the Wild West, it’s really great.
DAN: What do you think about changes in technology? What changes in technology need to happen in order to really start unifying all this data? Are there changes? Or is this something we can, you know, launch into tomorrow and just roll up our sleeves and you force your grad students to work on it for another week? Or do we need some kind of specific advance to to start working there?
ROGER: I think there are certain areas where we need centralized efforts at unification. So some obvious scenarios, and some of these have already been or are already under development. So a really great case in point is the MiBIG database which describes all of the biosynthetic gene clusters that are in the published literature. So that’s run by Marnix Medema and his team at Waginingen. And they have been extremely successful there because they brought together a consortium of researchers in the field. And they were able to define standards for the minimum information that should be reported. And to develop a schema by which you can report that in a reasonably standard way. And then the MiBIG repository is able to accept that standardized data. And they put a lot of energy behind the scenes into making sure they keep on top of the literature and that they capture gene clusters as they’re published and then curate and enter those which are not deposited.
DAN: Invaluable resource.
ROGER: It’s an amazing resource, and it’s a really touchstone example of how consortia like that can build tools which are of high quality and very stable. Some data types are easier to manage than others. NMR is actually – both NMR and mass spectrometry are a little bit more difficult to handle. The number of variables is quite high. Mass spectrometry, in particular, the number of different ways in which you can analyze the same sample is frighteningly large. And so there are two problems there. One is that even though your molecule may be deposited in a repository, if the acquisition conditions are very different than the ones under which you analyze your sample, then the comparison may not be that relevant, but also the amount of information you need to capture and the ways in which you choose to standardize that are… that’s quite tricky. And again, industry has put a lot of effort into trying to sort that out. But it means that it remains quite difficult to relate databases and data sources of different types to one another, which is ultimately what we need to do as a field.
What we’d really like is a central repository, which has all the structures and their corresponding gene clusters, and their MS and MS-MS data under a huge range of different acquisition conditions and their raw NMR data, and their bioactivity data and so on and so on. That data set does not exist and will be hard to do. I think we can do this, some of that in pieces. And some of those relationships are already being built. But it is going to be the challenge of our age, I think.
DAN: Yeah, yeah. Definitely an aspirational goal, and something that needs to happen. But…
ROGER: Yeah, that will be quite difficult. I would say the other place where we’re going to see large and wholesale change is in data analysis strategies. So we’ve seen in the last 15 years, enormous advancements in hardware, both on the NMR side and the mass spec side. So the single biggest advancement in NMR was the development of cryo probe technology and the improvement in sensitivity of those instruments. And that’s been followed by developments in pulse sequences and improvements in the ways in which you acquire data. Mass Spectrometry has also had an enormous, sort of, sea change. In terms of the hardware. So when I was a grad student, the mass spectrometer was in the basement behind – what do you call those things – like, a counter. So when I was a grad student, the mass spectrometer was behind a counter in the basement, and it was run by a dedicated team. It was the size of a hotel room, and it was always broken.
DAN: I remember Yeah.
ROGER: And now we have an ion mobility QTOF instrument in the lab, which our undergrads run. And the difference there in reliability, accuracy, sensitivity, all those things have changed beyond all recognition. What has not yet kept pace are our data analysis strategies. I think everyone who does, particularly, mass spectrometry – if you do a lot of mass spectrometry, everybody will bemoan the challenges associated with data analysis, and particularly, with jumping between instruments or jumping between methods. There is an enormous amount to do there. I think there is – I still – I think we still throw away a huge amount of valuable information in mass spectrometric datasets because of our inability to extract all the value from them. And I think that’s where we’re going to see big change.
ALISON: Yeah, just to follow up on that, like how… What other data analytic approaches could people use? Like, how could people innovate in that area?
ROGER: Well, I think that again, when I was a graduate student, the mass spectrometer was the last thing you used. So you would purify a metabolite. You would solve its structure by NMR. And when you had it clean, and you were pretty sure you knew what it was, you’d get a mass back in order to verify the formula and satisfy the requirements for submission to the journal. Now, because of the ready availability of data from mass spectrometers, it’s been used as a sort of front end tool, so it’s being used as the first stage in discovery.
There are so many different ways that you can look at even a simple mass spectrometry experiment and derive information from it. So, the accurate mass, the isotope distribution, the collisional cross sectional area, if you’re doing ion mobility, the retention time if you standardize those, the MS-MS or MSn fragmentation patterns, in principle, the isotope patterns of those fragments depending on how you acquire the data. The data goes down and down in layers and layers and layers. And this comes back to this question about whether you know about the existing canon of structures or not.
So, if you have that huge array of different pieces of information from a single mass spec experiment, and you have a fixed pool of things which are known, then the question becomes: “Is this metabolite any of these previously known metabolites?” and there is enough information there that I think we ought to be able to do quite a good job of answering that. It requires development in lots of areas, we would like to do a better job of understanding gas phase reaction mechanisms.
We would like to do a better job of predicting MS-MS spectra, so on and so on – interpreting fragments, all this sort of stuff. So I think there is a huge opportunity there. But it requires developments on the software, on the technical side, and also developments in basic science. I think we don’t know enough about gas phase chemistry yet. We don’t know enough about fragmentation mechanisms, and prediction of those, the way those things work. And so I think there are opportunities across the spectrum from those who are doing very fundamental research, at the sort of basic building blocks of how mass spectrometers operate, and how analytes are produced, right through to applications and how you use that information in the most efficient and the most successful way. So, yeah, there’s room for everyone.
ALISON: So if I can just see if I understood that. Improvement in data analysis would look like just having a better understanding of how these, I guess, the mapping of the masses and the ions to structure in a fundamental way. Like that we don’t understand so much about the chemistry – about the way that these molecules can break down – to interpret the spectra and that innovations in that area would be really helpful.
ROGER: Yeah, I think that’s exactly right. I think understanding what happens to molecules during the process of analysis is an area where … we’re still not – we don’t have a perfect understanding. And then there are practical issues as well. We are still not particularly good at differentiating real analytes from noise, and thinking about best practices for experimental design, and which is the best instrument for a particular experiment? Is it worth doing replicates? What do you do about variations in concentration? How do you design the experiment so that you have the clearest possible picture of the chemistry that you’re trying to analyze? I think that, at the moment, there are still a very wide array of different approaches. And I suspect that we will start to see a sort of collapsing of those approaches as time goes on, as some strategies win out as the most accurate and the most informative. And it will be interesting to see that development, I don’t know where that’s going to go, but it’ll be interesting to see what happens.
DAN: So I guess the last thing I wanted to just ask is: Now that, you know, you’ve got all this infrastructure built, surely, there was a reason that you did this in the first place. So what are you going to use all of this to actually do in your research? [long pause] Or are you an infrastructure guy now?
ROGER: No, no.
DAN: I’ve never known you to be!
ROGER: We’ve now made a commitment to supporting the infrastructure, which is also a bit daunting! But no, we absolutely built it in order to to pursue fundamental questions in natural product science. I would love to know, you know, what are all the natural products that we can possibly find? Does the building of this data set allow you to say anything about what the scope of natural products diversity is likely to be in the environment? Can we use this broad-scale view of the chemistry in order to start to make predictions about environmental roles of these compounds? Can we perform studies such as looking at the distributions of different classes of compounds? So for example, if you see Class A, do you always see Class B and Class C? And if so, do they pay play some complimentary role in the environment? So you can imagine using the data in a very broad sense like that. I could envisage projects where you say, “Okay, I see, this group of organisms has this set of molecules. And we know that to be successful in the environment, organisms need molecules that do these kinds of things. And I know that some of the molecules I’ve identified do some of these jobs. Therefore, some of the molecules which don’t yet have jobs must most likely play one of these other roles.” So if we’re still missing a siderophore, we should look carefully at these as being candidates siderophores. Or for cell-cell signaling or for, you know, other other roles here. So I could imagine that once the set is complete, you can start asking much broader questions about how chemistry is diversified in nature, and when it is not diversified and why? Where are the holes? Which bits of chemical space are you never going to find in nature? And are any of those bits of chemical space relevant to human health? You can imagine that compounds found in nature are produced by organisms for their own benefit. But that’s not necessarily relevant to many of the diseases that we seek to treat. So, it may be that molecules which are natural product-like, which would actually be very beneficial in hard to hit target areas, are never going to be found in a classical natural products program because they are selected against because of their lack of function. So knowing about the full sweep of chemistry which is out there allows you to identify obvious holes and then use semi-synthesis or synthetic methods in order to target and go after these sort of new areas of chemistry in a very directed way, which is still inspired by nature, but maybe builds on what nature has to offer. So, lots to do.
DAN: Roger, those are fantastic questions, and I’m looking forward to you generating the answers.
ROGER: Well, let’s see. I think it’s gonna be a worldwide effort. But it’ll be fun to see how people use the data in different ways.
DAN: All right. Thanks so much for talking to us.
ROGER: It’s my pleasure. Thanks very much.
DAN: I’m Dan Udwary, and you’ve been listening to Natural Prodcast, a podcast produced by the US Department of Energy Joint Genome Institute, a DOE Office of Science User Facility located at Lawrence Berkeley National Lab. You can find links to transcripts, more information on this episode, and our other episodes at naturalprodcast.com
Special thanks, as always, to my co-host, Alison Takemura. <woohoo> If you like Alison, and want to hear more science from her, check out her podcast, Genome Insider. She talks to lots of great scientists outside of secondary metabolism, and if you like what we’re doing here, you’ll probably enjoy Genome Insider too. So, check it out.
My intro and outro music are by Jahzzar.
Please help spread the word by leaving a review of Natural Prodcast on Apple podcasts, Google, Spotify, or wherever you got the podcast. If you have a question, or want to give us feedback, tweet us @JGI, or to me @danudwary. If you want to record and send us a question that we might play on air, email us at [email protected]. And because we’re a User Facility, if you’re interested in partnering with us, we want to hear from you! We have projects in genome sequencing, DNA synthesis, transcriptomics, metabolomics, and natural products in plants, fungi, and microorganisms. If you want to collaborate, let us know! Find out more at jgi.doe.gov/user-programs.
Thanks, and see you next time!