In this episode, Dan and Alison discuss the basics of genome mining, as we get ready to talk to some of the experts in this area of natural products research. We talk about the California Gold Rush (it’s relevant! I promise!), talk about how genome sequencing has changed genome mining over the years, and we talk about a new publication out of JGI in Nature Biotechnology, for which Dan conducted genome mining on thousands of metagenome-derived genomes. Check out http://naturalprodcast.com for show notes with links to papers and other learning resources.
Relevant links
DAN UDWARY: You’re listening to the Department of Energy Joint Genome Institute’s Natural Prodcast, a podcast about natural products and the science and scientists of secondary metabolism.
Welcome back for our second season of Natural Prodcast. This first episode up is another primer episode. These are episodes where Alison and I talk about a topic or kind of a subdiscipline in the natural products or secondary metabolism field. Hoping to do these every once in a while, especially when we start talking to people who specialize in that area.
In this primer, we talk about my favorite topic in, arguably, my area of expertise, which is genome mining. You’ll hear us talk about what it is, hear me make a pained analogy to the California gold rush. And we’ll talk about a new JGI paper that’s just come out where I got the chance to do genome mining on more than 50,000 genomes that we derived from meta-genome sequences.
As always, you’ll find transcripts and show notes with links to lots of other resources, especially for these primer episodes, if you want to dive deeper on all this at http://naturalprodcast.com. And then coming up after that, we’ll have a lineup of international scientists who also do genome mining. We’ve got a great, fun bunch of little shows lined up, and I’m really looking forward to getting them out to you all. But for now, here is episode 10 of Natural Prodcast, the primer on genome mining.
Hey, Alison.
ALISON TAKEMURA: Hey, Dan.
DAN UDWARY: So I guess this is now– we’ll call this the second season of Natural Prodcast. We’ve both taken a little bit of time off. Genome Insider still has been coming out, but I have been a slacker. We’ve had all kinds of chaos here between the pandemic. I had an international move. You had an interstate move. We’ve had forest fires here in California and, uh–
ALISON TAKEMURA: I had snow.
DAN UDWARY: You had snow already. Yeah, that’s crazy.
ALISON TAKEMURA: Yeah.
DAN UDWARY: I wish we had snow. And [laughs] kids back in school from home, not back at school. So, yeah. So there’s been a lot going on. But Natural Prodcast needs to come back, right?
ALISON TAKEMURA: That’s right. Now is the time.
DAN UDWARY: All right. So I thought we’d kick things off this time around, talking about a subject that’s near and dear to my heart. And that is, we wanted to do a primer episode on genome mining. So what do you know about genome mining?
ALISON TAKEMURA: I know that it’s looking in the genome for genetic elements of interest, you know, something like treasure.
DAN UDWARY: There you go.
ALISON TAKEMURA: It involves treasure, yeah. [chuckling]
DAN UDWARY: So I’ll tell you, when I do these, I try to picture myself talking to– and this is not, in any way, meant to diminish you. This is just how I’m picturing this in my head.
ALISON TAKEMURA: I’m already insulted.
DAN UDWARY: You are a trained scientist with a PhD. I know that you know what you’re talking about.
ALISON TAKEMURA: A long time ago.
DAN UDWARY: But when I picture these, I picture myself talking to my mother, trying to explain science to my mom. So I always try to, when we do these definitions, try to keep the simplest possible thing to explain it.
So I think when I think about what genome mining is, if I want to explain that to someone who doesn’t know much about genetics or anything else, science wise maybe, what genome mining is, then, is looking at DNA sequence and trying to find the things that you want to find, things that are of interest.
And usually, in terms of bacteria and secondary metabolism, those things that we want to find are medicines. Medicine has always been the main driver for secondary metabolism drug discovery. Because we always need new antibiotics. We need new cancer agents. We need treatments for humans. And it just so happens that bacteria make a lot of the kinds of molecules that humans find useful as drugs.
So yeah, so genome mining, that is looking at the DNA sequence to try to find those things. So, the DNA sequence isn’t itself medicine, but the coding within the DNA codes for proteins that do the chemistry that put molecules together that become bioactive molecules in some cases, right?
ALISON TAKEMURA: Right, right, got it. And just thinking about it from I know the JGI perspective here at the Joint Genome Institute, because we don’t work on human health or on medicines per se, how do you see genome mining and looking for secondary metabolites in the genome as relevant to the JGI mission?
DAN UDWARY: Yeah, that’s a really good question. I think that’s something we sort of covered in the other primers. So people should go back and listen to those. But yeah, the mission of the Joint Genome Institute is under the DOE’s mission of energy and environment.
And so we know that lots of different types of organisms make lots of different types of molecules, not just things that we would be interested in as medicines, but also things that are important in their own environments. Like, bacteria make antibiotics in order to challenge or kill off competition, right?
And plants do the same sorts of things. And then, bacteria often live in symbiosis with plants and might actually be producing molecules that are helpful to the plants. And so, understanding those broader environmental and ecological roles for secondary metabolites is what our mission is. What’s important to us.
ALISON TAKEMURA: Yeah, and actually, that makes me think about how we can kind of use an extended metaphor. And not only are we interested in medicines for people, but also those molecules that mediate a well functioning ecosystem and environment and earth.
So yeah, it’s like these molecules can just mediate so many kinds of interactions. And by understanding them, we can promote the health and well-being of not only individual organisms, but collective organisms.
DAN UDWARY: That’s right. You’ve got it, yeah. So to bring it back around… So we might not be interested in, say, the drug discovery mode of genome mining. But I do do a lot of genome mining here at the JGI. That’s one of my main tasks. And I wanted to explain a little bit what that means and how we do it here and maybe give an example of a recent publication.
ALISON TAKEMURA: Awesome. Yeah, let’s dive in, Dan. Where does the genome mining metaphor even come from?
DAN UDWARY: Sure, so it’s kind of an older term now that comes out of the way that people were doing– the way that people were trying to locate and sequence and get the information on biosynthetic gene clusters. And I’ll explain all this.
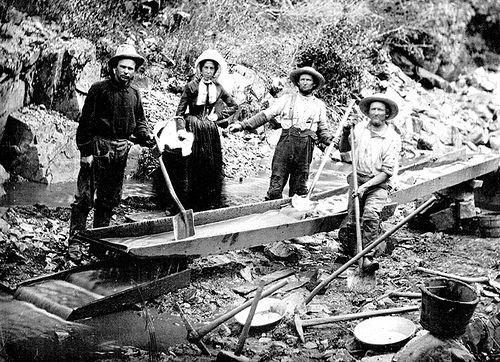
So but first, let’s talk about gold mining. So we’re here in California. And gold mining was a big endeavor. And I’m no expert, but the way that I understand this is, the way this worked is people who were trying to find gold would go out into the hills. And they knew from example that gold is a soft metal and that if there were gold somewherenearby in the nearby hills, then you could determine that by finding runoff.
So you would go to the streams where the hills, as it rained or whatever, any water that washed down off the hills might carry small pieces of gold along with them and end up in the beds of little streams. This will all become clearer as we get into the genomics. So–
ALISON TAKEMURA: I’m just enjoying the ride right now. Go ahead.
DAN UDWARY: So you would start by panning for the gold in the streams. And when you started finding– you would go up and down the stream, and you would find places where there might be little flecks of gold. You had some idea, then, that there was gold nearby.
And so then you’d start cordoning off areas and digging more and then trying to locate the gold. And eventually, hopefully, if you got lucky enough, you would find a vein of gold. Because gold, the way that– I don’t know– it deposits, I guess, it deposits in veins. And so if you find little pieces, you know that there are probably going to be big pieces somewhere, right?
The National Park Service has a great writeup on the kind of gold mining Dan is describing!
And so it turns out that secondary metabolism, genetically, kind of works the same way for reasons that are still mostly unclear. And again, we talked about this in the earlier primers. In bacteria, at least, and in most fungi, biosynthetic gene clusters, which are the genes that do the biosynthesis of a secondary metabolite, those are clustered together in a genome for some reason.
So if you could find a little piece of a gene that you knew was probably biosynthetic that encoded for some secondary metabolism, then you would probably, if you could search the area, you would find the rest of the biosynthetic gene cluster. And so we’ve learned a lot about how biosynthesis pathways happen, just by being able to find one kind of gene and then looking at its neighbors.
So it’s really the same kind of thing. Before the age of genome sequencing, we had to basically break up the pieces of DNA of whatever organism we thought would have the biosynthetic pathway. And you break that up and clone it into cosmids, or fosmids, or whatever. And you would scan those pieces by hybridization or maybe sequencing if you were rich enough. [CHUCKLES]
And once you found a little piece of something that looked like it was a biosynthetic pathway, then you would sequence the whole thing, and you’d use that to then seek out the other pieces of the pathway. And so it was very, very analogous, actually, to gold mining. And so genome mining became the term that really kind of fit and stuck.
ALISON TAKEMURA: Cool. And so how did you get involved in genome mining?
DAN UDWARY: Oh, well, OK, sure. So back in graduate school, I worked in Professor Craig Townsend’s lab. And I hope we get Craig on here at some point. I’ve opened the door for him, and he’s a busy man. But he was working on aflatoxin biosynthesis. And so when I came into graduate school, I worked on that project.
And it was working exactly that same kind of way, where we had– aflatoxin is produced by a fungus called Aspergillus. We were mostly working in Aspergillus parasiticus. Yeah, so we were sequencing the pieces of that and trying to understand that biosynthetic pathway in exactly that way, sequencing little pieces of it, finding the pieces that attached to the other pieces, and sort of building out the puzzle from there to find that vein of gold.
Not that aflatoxin’s a particularly good drug, but it has a really interesting biosynthetic pathway because fungal genes are cool. And if you like fungal genes, you should go back and listen to the Nancy Keller interview, where we talked a lot about that.
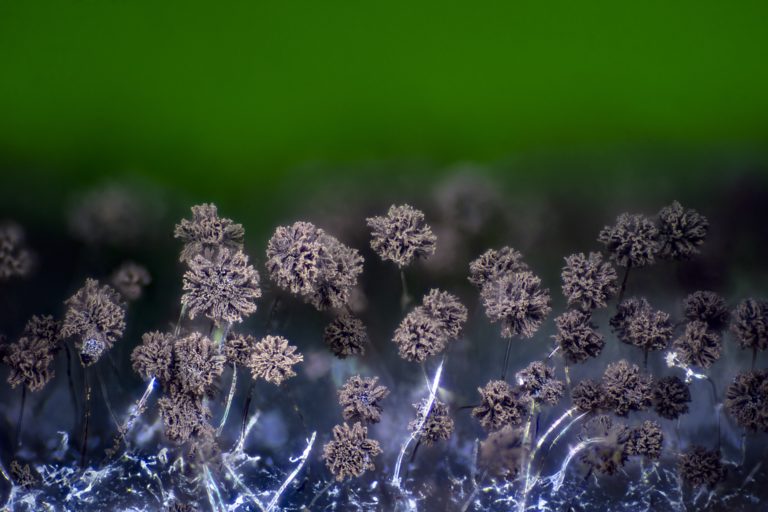
A nice map of the Aflatoxin biosynthetic gene cluster and its reaction pathway can be found in Yu et al, APPLIED AND ENVIRONMENTAL MICROBIOLOGY, 2004, 1253–1262, 70:3
ALISON TAKEMURA: And so did you succeed in finding your vein of gold with aflatoxin?
DAN UDWARY: Oh, yeah. Well, yeah. When I came into the lab, it was already basically there. We were just seeking out the last few pieces and trying to understand the enzymology. So we would actually express the genes and do the biochemistry to understand what the biosynthetic conversions were and what the mechanisms enzymatically were.
ALISON TAKEMURA: Where did you see that research or those results heading? Toward an application, toward just better understanding fungal biosynthesis?
DAN UDWARY: Yeah, this was really mostly to understand some really complicated enzymatic conversions. The last couple of steps and the first couple of steps of aflatoxin biosynthesis are really unusual. They’re oxidative conversions and do some really complicated carbon skeleton rearrangements, chemistry that we probably don’t [need to] get into. But yeah, it was for enzymology.
Yeah, so genome mining really hinges on the fact that biosynthetic pathways are clustered, that the genes are next to one another. And that’s basically universal in bacteria. There are a few exceptions, but almost always the genes are clustered. Like I said, most of the time, at least the ones we know about, are clustered in fungi.
Plants are a different story and much more complicated. And there’s a reason we don’t understand as much about plant biosynthesis as we do in bacteria. But really, because it’s easy to find biosynthetic pathways in bacteria. As you might expect, bacteria are the most well investigated.
So most of the things that I think we’ll talk about in terms of genome mining mostly apply to bacteria with the understanding that more complicated organisms, things get more complicated and don’t always work right. But yeah, bacteria are easy nowadays to sequence.
The other thing about bacteria is that they are easy to propagate and store. They’re easy to grow up, easy to throw in a freezer. Most of the successful genome mining has been in the antibiotic space. And it just so happens that bacteria are the best sources for finding antibiotics because bacteria produce antibiotics to kill their competition so that they can survive in their ecological niche.
ALISON TAKEMURA: Yeah, bacteria sound like a good place, to me, to look for diversity because there are so many bacteria, because it is so easy to sequence their genomes comparatively to especially plants. So yeah, so from there, kind of, you’ve laid out this foundation for us– what organisms people use for genome mining, why it’s called genome mining, how the biosynthetic gene clusters are clustered together so it can be easy to find the whole pathway. So how do we do genome mining now? What does that process look like?
DAN UDWARY: Yeah, it’s a little bit different now, just because of the ways that we can generate data. So, obviously, it’s very apparent to us here at the JGI, but genome sequencing is pretty routine nowadays, right? And so, generally, really almost always now, we have the genome sequence before we have almost any other information about many organisms.
In a lot of cases, certainly in older times, someone would have really done a somewhat exhaustive chemical analysis of a bacterium that they’re interested in, usually some sort of activity screens to try to find some bioactivity that they were interested in. And then, from there, they might do genome mining to try to understand the biosynthetic pathway or to help identify the compound that they were interested in.
But nowadays, really, people are sequencing before they really do anything else, just because it’s so cheap and fast and gives you so much more information to build on. So, yeah, so genome mining is really just looking at the sequence and trying to identify what’s there before you even necessarily know what’s there.
So the way, then, that we try to find biosynthetic gene clusters in genomes is by homology search. We’re building on the backs of people who’ve done a lot of work over the years. We have a pretty good idea of what the different families of secondary metabolism pathways look like. We know what the genes are.
And often, we’re just looking for relatives of those kinds of genes. So we know what a polyketide synthase looks like. We know what a non-ribosomal peptide or a ribosomally processed peptide looks like. And so we take genes that we think are going to be similar, and we do a homology search. We compare those sequences with our sequence.
The premier tool for that these days, the one that pretty much everybody uses, is called antiSMASH. And it is another community effort that’s been built up over the years and is really strong for finding lots of different things of the kinds of things that we know about.
(The online version of antiSMASH can be found here. It also has versions specialized for fungal BGCs and for plants. As an open source project, you can also download the code and run it on your own computer, or as a Docker container or Anaconda package.)
ALISON TAKEMURA: I just wonder if that name, antiSMASH, was in part inspired by Super Smash Brothers. Because I know that that’s a very popular Nintendo game. And I just hear a lot of friends, like, oh, do you want to play Smash? And so–
DAN UDWARY: Yeah, it’s– [laughs]
ALISON TAKEMURA: That could be an origin story.
DAN UDWARY: That’s really reaching. No, antiSMASH is the Antibiotics and Secondary Metabolites Analysis Shell. I don’t know. It seems like a pained acronym, but there you go. [laughs]
ALISON TAKEMURA: All the more reason why it could have been inspired by Super Smash Brothers.
DAN UDWARY: I don’t know about that, Alison. [laughs] It’s definitely reaching.
ALISON TAKEMURA: All right, I’ll just go with my theory. OK, continue.
DAN UDWARY: Yeah, and so, a lot of us were doing these kinds of homology searches, but antiSMASH took, basically, all of the known things that were out there and created some good rule sets and put it all together in one package so that it opened up secondary metabolism identification to anyone who wanted to do it. And it’s been really, really valuable for the community.
ALISON TAKEMURA: So it’s still a little vague to me. What do you mean by putting a bunch of tools in one package?
DAN UDWARY: Oh, sure. So if I wanted to find a polyketide synthase, then I would take a couple of the domains, which are small pieces of the sequence of a polyketide synthase. Each domain catalyzes some specific reaction. And so I would take a collection of those.
And I would either use blast or hammer, which are common homology search tools. And I would blast those against the genome that I wanted to search. And if I found a place in the genome where there were a bunch of those hits all in the same place, then I would have a pretty good guess that there was a biosynthetic gene cluster there.
But that’s one family of biosynthetic genes. And so, I think antiSMASH– oh, I should actually have the number. But I might have to edit it in.
ALISON TAKEMURA: Oh, you can do that later.
Dan insert: He did not
DAN UDWARY: I don’t even know where to find it fast. [LAUGHS] But antiSMASH takes lots of these. I know it uses about 200 different families of genes that it searches for and uses a rule-based system that classifies the things that it finds into which biosynthetic gene cluster family. So it will find all of your polyketides, all of your non-ribosomal peptides. It’ll find all of your terpenes and RiPPs and all kinds of other things that are even more obscure.
ALISON TAKEMURA: So, yeah. So it puts all those things together. That’s pretty remarkable. It does definitely sound like a huge service to the secondary metabolite community. What other techniques are there for finding these biosynthetic gene clusters?
DAN UDWARY: Well, there really isn’t too much else. So antiSMASH has been sort of a good aggregator of different methods that people use when they’re doing homology searches. But it’s a rule-based method, and so you’re only going to find the kinds of things that you know about.
So there are a few other efforts you see sometimes. So pretty recently, Merck put out some software called DeepBGC, which is a machine learning tool that tries to look at patterns of Pfams through a genome and predict where regions of secondary metabolism should be. That’s still largely homology based, but then you’re using kind of a black box for the rule set.
(Like antiSMASH, DeepBGC is an open source project and can be downloaded here, or, better yet, installed as an Anaconda package.)
And so that does bring up– it does find things that maybe you don’t expect to be secondary metabolism and maybe a few false positives, too. But it’s a good effort, as a next step.
And I should also point out a tool called evomining, which takes a collection of sequences and tries to identify– to look for things that are sort of unique to the genomes, rather than specifically biosynthetic pathways. So it’s another interesting approach. Probably other people will be doing lots of things to try to find new ways to approach this problem.
Evoming is also available as downloadable code, or as a Docker container.
ALISON TAKEMURA: It reminds me of some research I heard about actually at JGI involving– could you continue to elaborate more on maybe an intuitive way of grasping that?
DAN UDWARY: Well, I’m thinking it’s a really tricky problem because secondary metabolism genes are duplications of primary metabolism genes. They have evolved together or been modifications and duplications of primary metabolism. And so being able to pick out things that are primary metabolism versus secondary metabolism when the genes are similar is a big fuzzy gray line.
And so, the things that we know about, the things that we can definitely identify because we have lots of examples of them, are the things that we find the most. And then there are probably dozens, hundreds of examples of secondary metabolism in very common genomes that we just haven’t identified yet because they look too similar to other known primary metabolism pathways, or we haven’t found molecules being produced by the things.
And so we don’t know what specific patterns of genes to look for. And so that’s especially problematic in a case where we have all the sequence data but none of the chemical data. So in meta-genomic sequences, we don’t even have the organisms. And so you have no chemistry and no hope of getting to the chemistry. And so trying to really identify all the secondary metabolism from that is really tricky.
ALISON TAKEMURA: Right, I see. I have a better sense now of the shape of the problem, and then how machine learning, because it feeds on so much data, right, to learn features of interest. And so, because that it’s such a fuzzy gray line as to what genes might be in a biosynthetic gene cluster or just the original genes for primary metabolism, that machine learning really would help distinguish and predict novel gene clusters.
DAN UDWARY: Yeah, it certainly could. You can imagine lots of different approaches to sort of tackling this kind of a problem that don’t involve– I don’t know– traditional statistical analysis kinds of things that we would normally do as biologists, which is really just the brain dead kind of blasting for things [laughs and trying to see what comes out.
Yeah, it’s an area that could certainly use more work, but it also is only going to work if you have validation of the results. And we should probably get someone on here sometime to talk about isolation. Natural product isolation is not a trivial endeavor. Getting those molecules that are produced by a given organism into a tube in a pure form is not an easy task at all.
So you can make all the predictions in the world that you want, but until you have validated those predictions, it’s really hard to actually build on predictions as an input source. Garbage in, garbage out. So if we’re just using predictions to refine our predictions, we’re not really going to get anywhere.
ALISON TAKEMURA: Mm-hmm, yeah. Spoken like a good scientist.
DAN UDWARY: [laughs] Well, there’s realism. And so, this actually brings me [to] the idea of what we were doing at the company that I worked for before I worked at JGI. And that company was called WarpDrive, which doesn’t exist anymore, but most parts of it had been bought by Gingko. And I’m hoping we have some of those folks on here.
But what we did at WarpDrive was exactly in this kind of a vein. We sequenced in the end about 150,000 genomes, just to look for a very specific class of natural product. So we found lots of things, lots of examples. But then actually getting those things into a tube, and actually, then, even beyond that, having those things do what we were hoping that they would do, [laughs] Biology doesn’t really always work out that way.
ALISON TAKEMURA: I always tell my partner, we know nothing about biology. Biology hates us. It’s a little dark, but. [laughs]
DAN UDWARY: Yep.
ALISON TAKEMURA: It’s very mysterious.
DAN UDWARY: Even when we think we know exactly what’s going on, we definitely don’t. Yeah, so that’s really the trick, is you can find the things. And genome mining now, instead of being the thing that sort of clarifies the chemistry, genome mining is kind of a guiding post to understanding what the chemistry probably looks like. The sequence tells you a lot, but it doesn’t tell you nearly everything.
What I think a lot of people hope for is that at a certain point, there will be an inflection point. Right now, we have lots and lots of biosynthetic gene clusters. So, in the ABC portal here at JGI, ABC being the Atlas of Biosynthetic Clusters, which is a subsection of the IMG data portal, which is a larger portal for microbial genomics. IMG stands for Integrated Microbial Genomics. It’s one of the big data portals here at JGI.
So within ABC, we have something in the neighborhood of 400,000, last I checked, biosynthetic gene clusters. And that’s a lot of biosynthetic gene clusters, so that should be a lot of chemical information, right? Well, not so much because we actually don’t know what the vast majority of those do.
There’s another data portal out there that’s not JGI’s. It’s called MIBiG, which is the database that has the “minimum information about a biosynthetic gene cluster”. And MIBiG has all of the experimentally characterized gene clusters with all of the information that they know about them. And so, there’s only about 2,000 entries in that. So we clearly, unambiguously know about 2,000 biosynthetic gene clusters out of ABC’s 400,000. So that is not a huge proportion. That’s, what, half a percent. And so there’s still a lot more work to do to tie gene clusters back to chemical products.
But the more we understand, the more we’ll be able to make better predictions. And so, I think a lot of people hope that, at some point, we’ll know enough that there’ll be an inflection point, and we’ll be able to look at a gene cluster and understand exactly what it would be doing in its biosynthetic pathways, or at least, to a better margin of error and to be able to tie up– this is the flipside. This is what we talked about with Roger.
Roger Linington has the NP Atlas that he is guiding. It’s a community effort of putting together the natural product structures that are known. I forget how many structures are in NP Atlas, but it’s a significant number of thousands. And if we’ve got a couple hundred thousand biosynthetic gene clusters, at a certain point, we’re going to know enough about how things work in both directions in terms of how a biosynthetic cluster makes a product and how a product should be synthesized based on its structure, that we should be able to tie those things together.
And so once we can do that, I think, then, things start to take off really fast. That we’ll better understand the biochemistry because you’ll be able to look at, in the same kind of way that you can identify a biosynthetic gene cluster based on knowing about a certain portion of it, once you look at the rest of the biosynthetic cluster, often, that tells you about the rest of the chemistry. It would be the same kind of thing if we could tie a molecule to a cluster.
We might know most of what the biosynthesis should be, but then there will be a couple of genes in there where the light bulb will go off, and you’ll understand, oh, those things are doing this. And then there will be analogs to those things that are in other biosynthetic clusters, and that helps you understand those better. And it builds. And that’s why I keep saying “inflection point”. At a certain point, we’ll have enough information that we can tie all that together and really kind of put all the pieces together in a better, more understandable way.
I don’t know how fast that comes. That could be a couple of decades away. But things are moving faster all the time. And a classical example of when this happened was when people started understanding NRPS A domains.
(Dan’s favorite review on NRPS structural biology is here)
And so we talked about non-ribosomal peptides a minute ago. Non-ribosomal peptides, remember, are big, complicated enzyme systems that really just exist to pull an amino acid out of solution and stick another amino acid or multiple other amino acids onto it to create a small peptide.
So at a certain point, a couple of different groups noticed that they could take all of these adenylation domains. And adenylation domains are the part of the NRPS that recognizes the amino acid that it’s going to incorporate, and the thing that actually pulls it out of solution and brings it into the rest of the enzyme.
Those A domains, you could line them up, and you could see that there were certain patterns of amino acids in the active site of the protein that would lead you to basically putting these things into different groups. And so, once you knew enough NRPSs – you need to know what amino acid was incorporated – you would know what that pattern of amino acids in the active site looks like, and then you could characterize it.
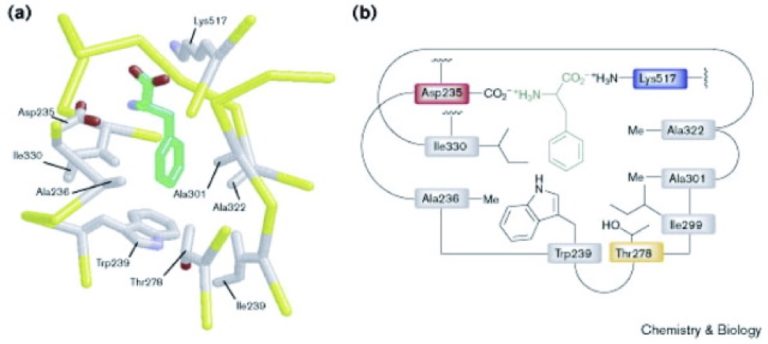
The classical study that demonstrated this is Challis et al, Chemistry and Biology, 2000, 211-224, 7:3
And so, you can then make predictions about NRPS’s that you hadn’t done any chemical experimentation on. And so now, nowadays, whenever we get an amino acid [Dan misspoke. He meant to say “NRPS”], you can basically usually predict, within a certain level of certainty, exactly what peptide is being produced by a non-ribosomal peptide. And so the point being, hopefully, we can do that with more classes of natural product gene clusters over time. That we’ll understand all of these situations better, and we can make better predictions. Yeah.
ALISON TAKEMURA: Yeah, I think it makes sense. It’s like having a baseball glove or some kind of glove, and you’re comparing different gloves. And then if you get enough gloves, you can see like, oh, yeah, they fall into different categories, and they code for different shapes that you can hold in the glove. And those are your amino acid sequences. And so, then, if you just look at the thing that encodes the gloves, the sequence, then you can begin to infer what is that ultimate amino acid sequenced in the non-ribosomal peptide.
DAN UDWARY: Mm-hmm, that’s a really good metaphor, yeah.
ALISON TAKEMURA: Thanks. [laughs] It’s tricky. Like, where did the gloves come from? Well, they come from some nucleotides, some DNA.
DAN UDWARY: Yeah, no, that’s actually really, really fitting.
[laughter]
Womp, womp.
ALISON TAKEMURA: So, Dan, I heard that there is a big paper coming out, or maybe it’s just come out by the time this gets released. And that you got to do some genome mining for that paper. Could you tell me about the paper and what you did?
What we’re talking about here is a paper published in Nature Biotechnology, titled ‘A genomic catalog of Earth’s microbiomes’. (Citation: Nayfach S et al. A Genomic Catalog of Earth’s Microbiomes. Nature Biotechnology. 2020 Nov 9. doi: 10.1038/s41587-020-0718-6)
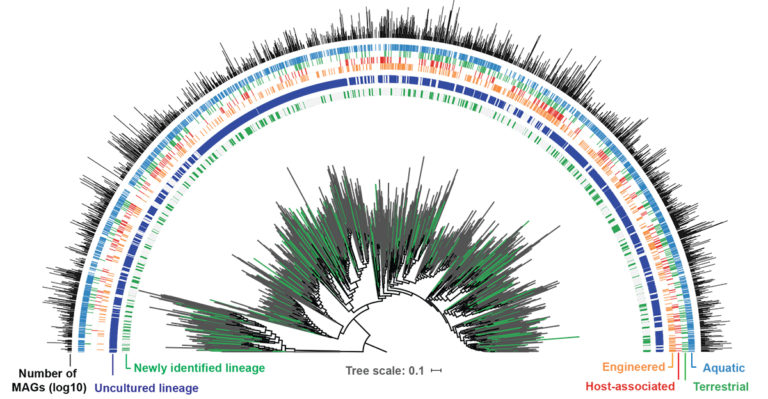
The GEM catalog expands the bacterial and archaeal orders as seen on the phylogenetic tree, with new lineages of uncultivated genomes from the GEM catalog (in green) and previously existing reference genomes (in gray). Around the phylogenetic tree, the strip charts indicate if an order is uncultured (blue; represented only by metagenome-assembled genomes or MAGs) or cultured (gray; represented by an isolate genome). The next four strip charts indicate the environmental distribution, while the bar plot indicates the number of genomes from the GEM catalog recovered from each order. (Stephen Nayfach)
DAN UDWARY: Yeah, I think that might be a good example of what we do, at least what I do here at the JGI and what can be done nowadays. So we refer to this as the GEM paper. GEM is the Genomic catalog of Earth’s Microbiomes.
And what this is, is a data set of bacterial and archaeal genomes that were created – these are Metagenome Associated Genomes, or MAGs. So these are genomes that were assembled from metagenomic sequence, metagenomic sequence being taking a sample of an environment and sequencing all the DNA that you can get from it, right?
And so these are, then, microbial genomes that we were able to assemble from that really larger set of data. And then this, as far as I’m aware, is the largest set of MAGs to date. Here, at JGI, we took more than 10,000 metagenomes, many of which were sequenced at JGI, and some were public data, and we put all of those together to produce 52,000 microbial genomes or MAGs.
And so, it’s a really big paper. There’s a lot of people that worked on it. It was a really large effort. But I got to do some of the secondary metabolism analysis on this. And–
ALISON TAKEMURA: Maybe before we get to that, can you–
DAN UDWARY: Sure.
ALISON TAKEMURA: –just give me a few examples of where these metagenomes came from?
DAN UDWARY: Oh, yeah. These things are sequenced from all over the place. So, lots of different environments, lots of different geographic locations. That’s one of the nice things about this data, is that we know exactly what environments they came from. So therefore, you can associate a specific microbial genome [as] being isolated from this particular source.
So we have very detailed environmental information often right down to the latitude and longitude, where it was sampled. And they come from all kinds of disparate environments. There’s some marine. There’s some soil samples. There’s human gut samples. There’s lots and lots of– animal associated, plant associated, just basically every environment that you can imagine that we have sequenced from is part of this data set.
ALISON TAKEMURA: Even the built environment?
DAN UDWARY: Yeah, there’s lots of those in there, too. Mm-hmm, sure. And so, when we got rolling on the secondary metabolism part of it, what I was hoping we would be able to do is maybe try to get some understanding of what kinds of biosynthetic gene clusters might be found from certain kinds of environments or certain kinds of species or whatever.
So for example, if you wanted to go find some unusual biosynthetic gene cluster, if you could pinpoint some environment where it was more common to find it, then you would have a good place to go sample from. So, yeah. So we did a bunch of that.
And it turns out that, as might be expected, these things aren’t perfect genomes in all cases. And the secondary metabolism biosynthetic gene clusters are often the things that suffer first when it comes to genome assembly. Some of the common biosynthetic systems – like a polyketide synthase or a non-ribosomal peptide synthase – they’re a little bit repetitive. And so they don’t end up being assembled properly or even at all in a genome. So those kinds of systems get very fragmented.
Another issue could be that in order to assemble these genomes, you go through a binning process. So you look at the DNA itself and try to make some assumptions about what kinds of organisms it might belong to and put them in separate bins and then assemble those pieces, kind of like if you were sorting your LEGO pieces by color, right? You’re going to put LEGO pieces together, you would first sort by color, and then you would attach them by color.
And so they try to do that with genomes. And that doesn’t always work for secondary metabolism because a lot of secondary metabolism is horizontally transferred. Things shift between even different genus. And so often, secondary metabolism genes don’t look like the genes in their host. And so, sometimes those kinds of things get lost in the binning process. And so, again, secondary metabolism ends up fragmented.
But, that said, we have lots of stuff. On average, we had something– I think we had two biosynthetic clusters per MAG, which is pretty good since a lot of human associated and certain environments just don’t seem to have organisms that tend to have a lot of secondary metabolism. A lot of it was fragmented, but we can still make some pretty reasonable assumptions about what kinds of gene cluster families are in which kinds of orgasms.
And so we have all of that information. We have 100,000 or really about 87,000 what looked to be novel biosynthetic gene clusters, like completely new things that we haven’t seen before. And so that’s really exciting.
ALISON TAKEMURA: Yeah, that’s amazing. 87,000?
DAN UDWARY: Yeah, 87,000 things that we couldn’t immediately say were the same as another biosynthetic gene cluster, at least a known one that had already been sequenced ever before. Yep.
ALISON TAKEMURA: I’m just trying to think of if there’s anything kind of in my normal day-to-day life where there are 87,000 kinds. What fraction of those things am I aware of? And I don’t know, maybe books in a library. And yeah, maybe I know 1%, or.
DAN UDWARY: Yeah, I mean, it’s a big proportion. If you go back to the ABC numbers where we had 400,000, we’re going to add another 87,000. So that’s, what, a 20%, 30% increase?
Dan insert: It’s a 21.75% increase.
ALISON TAKEMURA: Mm-hmm. OK, so what did you do once you found all of those?
DAN UDWARY: Well, so then we started taking that information and just looking at what kinds of gene clusters were found in what kinds of environments. And the surprise to me was that there wasn’t much to really point at. So we found that a lot of the human and animal associated genomes seem to have more Ribosomally Processed Peptides, or RiPPs. That sort of holds up with what we have seen in the past with people that have done metagenomic studies on, say, the human gut or whatever.
But other than that, there wasn’t really a whole lot of differentiation by environment. So it seems like you can find most things most places if you’re talking about broad biosynthetic groups. We didn’t really break it down by specific gene cluster families. We didn’t really have the technology to do that at the time. And that may be something we still explore. But mostly, yeah, what we find is that everything is everywhere, which is really kind of a weird surprise.
ALISON TAKEMURA: Yeah, that is weird. Why do you think that that might be?
DAN UDWARY: Probably the best explanation I could come up for it is that secondary metabolism is ancient and, therefore, widespread. So if secondary metabolism was there when– and it was. It had to be, right? There have always been– as long as there have been proteins, there have been duplications and modifications of those proteins to do some sort of slightly novel chemistry. That’s just how evolution works.
So if secondary metabolism is really ancient and most of the major families were there very early in terms of the existence of life on Earth, maybe when it was all waterworld, everything is swimming around everywhere and all mixed up, right? [laughs] And so everything ends up everywhere.
Just because a gene cluster is there doesn’t mean a gene cluster is active. And so the genes for things could be everywhere, but again, that doesn’t speak to the chemistry being everywhere. There could be very specific environmental circumstances of chemistry. You only turn pathways on when you need them or when you’re in certain environments. And so, we can’t really speak to all chemistry being everywhere. We can only speak to all genes being everywhere.
ALISON TAKEMURA: Hmm. Well, I think, I mean from an evolutionary perspective, I would think that genes that are no longer needed would end up being purged from populations. And that would go for chemistries as well.
DAN UDWARY: Yeah, that certainly seems to be the case.
ALISON TAKEMURA: And so, it could be that some of these genes you might be seeing them, they maybe are in process of declining in the population.
DAN UDWARY: That could be, too. I mean, that could be a good reason why we see lots of fragmentation. In a really more general population that isn’t selected for interesting chemistry, you’re going to find more fragmented biosynthetic gene clusters. And we can’t really speak to that either.
ALISON TAKEMURA: And then another hypothesis, I think, is that the gene clusters could be specializing, but in subtle ways that affect fitness. And so, doing a high level kind of analysis, you just might not be able to catch those differences. Everything might be everywhere, but only at a certain kind of coarse scale.
DAN UDWARY: Yeah I think that’s right. That’s why I say we didn’t get too deep into specific biosynthetic gene cluster families. I felt like with the fragmentation as it was, it’d be really reaching to kind of try to analyze that. But that’s definitely I think pretty likely. But yeah, still not yet proven. There’s a lot more work to do on all of this stuff.
ALISON TAKEMURA: Yeah, and if I were a grad student or an early career scientist looking to get involved in secondary metabolism more or even just starting to get involved, what might you recommend?
DAN UDWARY: Man, that’s a really big question and a really fun question– how do people get involved? So I think I’ve always had the perception that secondary metabolism is as broad as biology. I think anybody can get into it from any different angle with some expertise.
So we have DNA people and protein people and chemistry people and all the different kinds of people that we’ve already talked to. That there is an infinite number of more people with varying expertise in biology and chemistry that could really be participating in secondary metabolism [research]. And it would be very welcome because I think the field moves forward when different perspectives come in.
So I think knowing science and being skilled in some particular area of science can be applied to secondary metabolism almost always, no matter what that is. Almost always. How do people do that? I think just like any other aspect of science, you find a problem, and you start working on it from your particular perspective, and talk to other scientists, and get engaged. That’s how we do it.
ALISON TAKEMURA: Well, thanks, Dan.
DAN UDWARY: I mean, is that too pat an answer?
ALISON TAKEMURA: No, I think that’s a great answer. And I think it leaves a lot of space for someone who’s curious to get involved in whatever way that they might find an opportunity.
DAN UDWARY: Yeah, it’s out there.
I’m Dan Udwary. You’ve been listening to Natural Prodcast, a podcast produced by the US Department of Energy Joint Genome Institute, a DOE office of science user facility located at Lawrence Berkeley National Lab. You can find links to transcripts, more information on this episode, and our other episodes at naturalprodcast.com. Special thanks, as always, to my co-host, Alison Takemura.
ALISON TAKEMURA: Whoo.
DAN UDWARY: If you like Alison, you want to hear more science from her, check out her podcast, Genome Insider. She talks to lots of great scientists outside of secondary metabolism. And if you like what we’re doing here, you’ll probably enjoy Genome Insider, too. So check it out.
My intro and outro music are by Jahzzar. Please help spread the word by leaving a review of Natural Prodcast on Apple Podcasts, Google, Spotify, or wherever you got the podcast.
If you have a question or want to give us feedback, tweet us at @JGI, or to me, at @DanUdwary. If you want to record and send us a question that we might play on air, email us at [email protected].
And because we’re a user facility, if you’re interested in partnering with us, we want to hear from you. We have projects in genome sequencing, DNA synthesis, transcriptomics, metabolomics, and natural products in plants, fungi, and microorganisms. If you want to collaborate, let us know. Find out more at https://jgi.doe.gov/user-programs. Thanks, and see you next time.